Data-Driven Modeling for Additive Manufacturing of Metals: Proceedings of a Workshop
National Academies of Sciences, Engineering, and Medicine,Division on Engineering and Physical Sciences,National Materials and Manufacturing Board,Board on Mathematical Sciences and Analytics
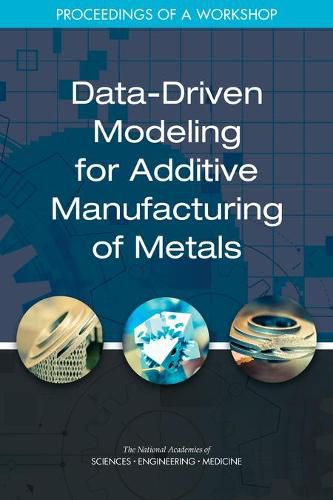
Data-Driven Modeling for Additive Manufacturing of Metals: Proceedings of a Workshop
National Academies of Sciences, Engineering, and Medicine,Division on Engineering and Physical Sciences,National Materials and Manufacturing Board,Board on Mathematical Sciences and Analytics
Additive manufacturing (AM) is the process in which a three-dimensional object is built by adding subsequent layers of materials. AM enables novel material compositions and shapes, often without the need for specialized tooling. This technology has the potential to revolutionize how mechanical parts are created, tested, and certified. However, successful real-time AM design requires the integration of complex systems and often necessitates expertise across domains. Simulation-based design approaches, such as those applied in engineering product design and material design, have the potential to improve AM predictive modeling capabilities, particularly when combined with existing knowledge of the underlying mechanics. These predictive models have the potential to reduce the cost of and time for concept-to-final-product development and can be used to supplement experimental tests.
The National Academies convened a workshop on October 24-26, 2018 to discuss the frontiers of mechanistic data-driven modeling for AM of metals. Topics of discussion included measuring and modeling process monitoring and control, developing models to represent microstructure evolution, alloy design, and part suitability, modeling phases of process and machine design, and accelerating product and process qualification and certification. These topics then led to the assessment of short-, immediate-, and long-term challenges in AM. This publication summarizes the presentations and discussions from the workshop.
This item is not currently in-stock. It can be ordered online and is expected to ship in approx 4 weeks
Our stock data is updated periodically, and availability may change throughout the day for in-demand items. Please call the relevant shop for the most current stock information. Prices are subject to change without notice.
Sign in or become a Readings Member to add this title to a wishlist.